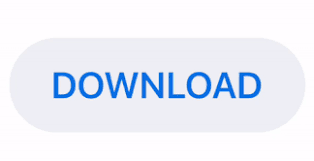
Often, these gaps come in the form of employees not having the right sets of skills to work alongside AI. "Organizations must be sensitive to the fact that implementing AI is a human as well as a technology change," Solotorevsky said.īecause of this, part of the AI implementation plan should be a gap analysis that identifies the workforce and workflow holes in an organization that must first be addressed before introducing AI. Yet, despite AI's potential to fight fraud, it's not easy for organizations to move to AI. "In a case study performed by TM Forum and Amdocs, Bell Canada experienced a 150% improvement in the time it takes to detect fraud losses in a significant portion of its fraud, and a 200% improvement in the time required to identify new fraud schemes," Solotorevsky said. SEE: Machine learning is a great tool for cybersecurity, but be cautious, expert says (TechRepublic)īell Canada's success with AI is one example. "For example, if a retailer can predict at the point of sale the propensity that a new customer who wants to buy a smart phone is committing fraud, it can take extra precautions, such as making an additional ID check of the customer." "Machine learning is also extensively used to create predictive future fraud models," Solotorevsky said.

These learning models then continually improve themselves for greater accuracy. By applying self-adaptive AI with machine learning, companies are able to detect and adapt to these ever-changing fraud schemes quickly."ĪI works by automatically generating tunable learning models that assess the results of past fraud predictions. "They change because once fraudsters identify that their methods and strategies are detected, they alter them…. "AI's adaptability is crucial because fraud patterns change all the time," Solotorevsky said. With ML, AI fraud detection models can adapt and create new rules for fraud detection, which in turn reduce the exposure to fraud and monetary losses. With artificial intelligence (AI), there is a new approach to fraud detection that not only reduces time to discovery, but limits the window for fraud losses.ĪI makes it easier to identify changes in known fraud patterns because it uses machine learning (ML).
#ARTIFICIAL ACADEMY GAME NOT WORKING MANUAL#
Unfortunately, manual investigations of fraud consumed considerable time before fraud patterns could be identified. In past practice, the telecom industry handled fraud complaints manually. SEE: Cheat sheet: Artificial intelligence (free PDF) (TechRepublic) "We also still encounter traditional usage-based fraud scenarios such as PBX or IP-PBX hacking, callback schemes, and abuse of weak networks and devices." "We mostly encounter payment and subscription fraud, identify theft/impersonation, account takeover, insider threats, and SIM swap," Solotorevsky said. Solotorevsky cited a 2019 survey from the Communications Fraud Control Association (CFCA) that found that two-thirds of respondents experienced an increase in fraudulent activities.

The total losses across the industry are staggering." "Today, close to 2% or over $1.5 trillion in yearly global revenue is lost annually due to fraudulent behavior. Gadi Solotorevsky, CTO at Amdocs cVidya, an AI solutions provider. "Fraud is a major consideration in the telecom industry," said Dr.
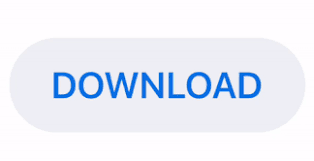